Artificial Intelligence
Improving AI Translation—A Leap Toward Global Inclusivity
Meta's translation tech is bridging the digital divides across 200 languages.
Updated June 6, 2024 Reviewed by Margaret Foley
Key points
- Meta's NLLB-200 expands translation to 200 languages, bridging digital divides and boosting inclusivity.
- This tech initiative uses advanced methods to support low-resource languages effectively.
- A benchmark shows a 44% improvement in translation quality, enhancing global communication and education.
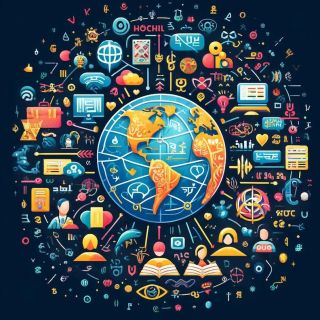
In an increasingly interconnected world, language barriers remain one of the most significant hurdles to global communication and knowledge sharing. While neural machine translation (NMT) systems have made substantial progress, their benefits have predominantly been confined to high-resource languages. The vast majority of the world’s 7,000-plus languages, particularly those with limited available data, have been left in the digital shadows. Enter the No Language Left Behind (NLLB-200) project, a new initiative designed to scale neural machine translation to encompass 200 languages, thereby ensuring no language is left behind—particularly in the context of little available training data.
Breaking New Ground in Machine Translation
The NLLB-200 project, developed by the NLLB Team, represents a significant leap forward in the field of machine translation. Traditional NMT systems rely heavily on large volumes of parallel bilingual data, which are abundant for high-resource languages like English, Spanish, and Chinese. However, for many low-resource languages, such data is scarce, resulting in subpar translation quality and exacerbating digital inequities.
To address this disparity, NLLB-200 leverages new techniques and architectures to create a unique multilingual model. The backbone of this model is the Sparsely Gated Mixture of Experts architecture, which allows for effective cross-lingual transfer and enables related languages to learn from one another. By employing novel data-mining techniques and a distillation-based sentence encoding approach (LASER3), the project effectively gathers and utilizes data for low-resource languages.
Innovative Approaches to Data Mining and Model Training
Data mining for low-resource languages presents unique challenges. The NLLB-200 team employed large-scale data mining to collect non-aligned monolingual data and identify semantically equivalent sentences across languages. This method ensures that even languages with minimal available data can benefit from the vast resources available in more common languages.
The training of the NLLB-200 model is equally important. By integrating a mixture of experts within the encoder and decoder layers, the model can handle multilingual data efficiently. Additionally, techniques like Expert Output Masking (EOM) and curriculum learning are used to counteract overfitting and enhance performance, particularly for low-resource languages.
A New Benchmark in Performance
To measure the success of the NLLB-200 model, the team developed FLORES-200, a comprehensive multilingual benchmark covering 40,000 translation directions. This benchmark, along with human evaluation metrics (XSTS) and a toxicity detector (ETOX), ensures that the model delivers high-quality, safe translations across all supported languages.
The results are impressive: NLLB-200 achieves a 44% improvement in translation quality compared to previous state-of-the-art models. This performance leap is not just a technical achievement but a significant step toward linguistic inclusivity in the digital age.
Starting New Conversations
The implications of NLLB-200 extend far beyond the realm of machine translation. By providing high-quality translation capabilities for low-resource languages, the project opens up new opportunities for education, information access, and cultural exchange. Students and educators from underrepresented language groups can now access a wealth of resources previously unavailable to them. Additionally, this increased accessibility encourages the creation and dissemination of localized knowledge, challenging the dominance of Western-centric content and fostering a more diverse digital ecosystem.
However, the journey does not end here. The NLLB-200 project highlights the need for continued interdisciplinary collaboration to address broader issues such as education, internet access, and digital literacy. While the model itself is a powerful tool, policy interventions and a holistic approach are necessary to truly overcome the structural challenges that many language communities face.
A Step Toward a Universal Translation System
In 2016, the United Nations declared internet access a basic human right, emphasizing the need for the unrestricted flow of information. The NLLB-200 project embodies this vision by breaking down language barriers and ensuring that no language is left behind. By making its models, data, and benchmarks freely available for noncommercial use, the NLLB-200 team has laid the groundwork for a universal translation system that is inclusive, equitable, and accessible to all.
As we look to the future, the continued development and refinement of multilingual models like NLLB-200 will be crucial in building a more connected and understanding world. With projects like these, we move closer to a reality where language is no longer a barrier but a bridge, connecting us all in the vast tapestry of human communication.