Education
A Primer for Evaluating Scientific Studies
A simple but useful set of criteria for assessing a piece of research.
Posted September 30, 2022 Reviewed by Vanessa Lancaster
Key points
- While not easy, it is essential for students and members of the lay public to evaluate relevant research studies.
- Some essential considerations are elusive because they have a subjective component or are technically complex.
- Useful criteria include statistical conclusion validity, internal validity, construct validity, and external validity.
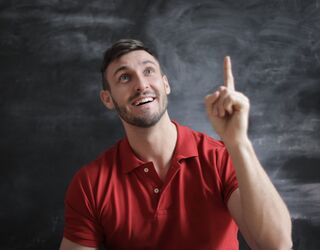
It is difficult for the non-expert to evaluate a piece of research. Often, such appraisals are based on misleading heuristics, such as catchy titles, endorsements by well-known figures, or wishful thinking about personal relevance and practical benefits.
There is no substitute for acquiring the necessary expertise, especially to inform important decisions you need to make. Learning to apply certain criteria can can be part of this self-education.
A point of clarification: My suggestions pertain to evaluating a single study. Questions about the overall support for a scientific claim across multiple studies are many, complex, and beyond the scope of this discussion.
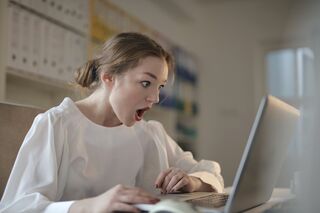
It’s interesting how important research differs from all the rest.
A former colleague often used a handy set of just three considerations: Are the findings interesting, important, and different? Good advice, to be sure. But there are limitations.
“Interesting” is subjective. What is interesting to one expert may or may interest others. Moreover, even where experts agree, what they find interesting may not interest the educated layperson, and vice versa. Not to say that the expert is always right about interestingness. Experts, like everyone else, have biases, which may distort their sense of what is interesting.
“Important” can be less subjective. Research claiming to have solved or paved the way to solving a major social problem may have more real-life importance than that which does not. Still, it can be hard to assess the potential for a research finding to have practical implications.
“Different,” in the sense of being novel, is in some ways the least subjective of the three. But that does not make it easy to apply. With the explosion of scientific journals and other outlets for research findings, not all of which are readily accessible, it can be hard to determine the originality of a piece of research.
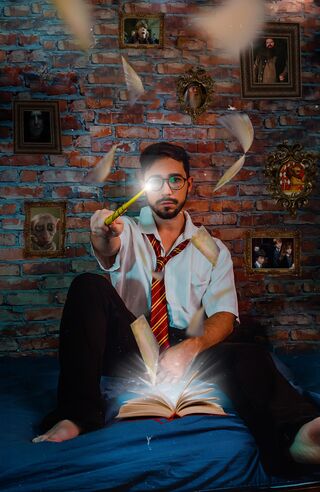
"It's just like MAGIC!"
A more elaborate set of considerations was designated by Robert Abelson using the nifty acronym “MAGIC.” “M” is for “magnitude,” or the size, in the statistical sense, of the observed cause-effect or correlational association. “A” is for "articulation," or the specificity or precision of the research. “G” is for "generality," or the findings's breadth of application or relevance. “I” is for “interestingness,” or the potential for the research to change how people think about the topic. “C” is for “credibility,” or believability.
Which is more essential: Eliminating threats or discovering opportunities?
Although “MAGIC” is certainly recommended, a more accessible starting point for non-experts can be found in classic papers by Donald T. Campbell and colleagues. It involves assessing whether a research study deals adequately with a set of threats to its validity. This may seem like a negative or adversarial stance, but the questions set up by this approach are intuitive, relevant, and hard to ignore.
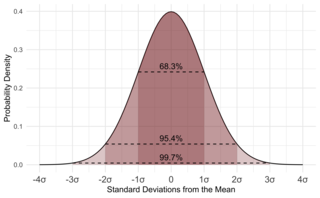
“Statistical conclusion validity,” in part, concerns the question of whether the observed relationship is statistically significant, that is, whether or not the data provide a basis for rejecting the null hypothesis, i.e., the hypothesis that there is no relationship.
It also asks the “magnitude” question with which “MAGIC” begins: “By how much, or to what degree, is the null hypothesis rejected?”
Null hypothesis testing has come under scrutiny recently, fault has been found with it, and alternatives have been proposed. But it is best to understand how it works and the basis for its strengths and weaknesses before moving on.
Statistics is not everyone's favorite topic. But the odds are (sorry!) that the more we understand statistics, the better off we are. In addition to the writing of Campbell et al., who provide excellent treatment of statistical and other technical details associated with validity threats, an undergraduate textbook is a very good resource well worth the investment.
For a study that reports differences between two or more groups of participants exposed to different treatment or conditions (e.g., an active drug vs. placebo), look into the t-test and Analysis of Variance. For a study that presents evidence for an association between two variables (e.g., body weight and life expectancy), look into the correlation coefficient r.
To distinguish statistical significance (the two groups likely differed; the relationship between variables probably was real) from the magnitude of the effect (How much difference/relationship was there?), start with the work of Jacob Cohen on effect size and statistical power.
“Internal validity” concerns the question of whether the relationship that has been claimed can be said to be causal or merely correlational. Most agree that casual inference is only supportable by well-conducted, randomized experiments. Here, random assignment to two or more experimental conditions creates substantial equivalence between groups so that any difference between them in the outcome of interest can likely be attributed to the causal effects of the different conditions.
“Construct validity” concerns the meaning of the concepts purportedly examined in the study. Did the measures capture the qualities or attributes they intended to reflect? Did any experimental treatments, manipulations, or interventions create or modify the states or conditions they intended to create or modify? Construct validity is a complex issue, and its details vary across research areas. One good place to start is with some excellent papers by Samuel Messick.
“External validity” maps onto the “G” in “MAGIC”: Are the findings such that they should generalize across different populations, settings, and measures and manipulations of the main concepts beyond those specifically involved in the study in question? Introductory statistics texts do a good job with the details of generalizability across different populations in their treatment of random/systematic sampling.
This consideration is also relatively intuitive: If a study sample is formed by selecting participants randomly from a well-specified population (or systematically, as in selecting every third member), it will closely resemble that population. Assessing generalizability to other settings and measures requires specialized knowledge of specific research areas.
The classic four validity threats defined by Campbell and associates provide an extremely useful framework for assessing research studies in psychology and other fields. Of course, its application raises questions, such as how exactly to evaluate each criterion and how the four criteria should be prioritized.
The latter question is a good way to generate discussion in a research methodology class; I come down valuing construct validity above the others; if we cannot be sure exactly what was measured or manipulated, where can we go from there? But much depends on the nature and importance of the research topic and the field's current state.
The threats-to-validity framework also leaves other questions, such as “Interestingness” and practical importance. But in many cases, those questions are moot if the work fails to address the four validity threats. Still, these are critical considerations, and the self-education needed to address them effectively is certainly worth the effort.
Copyright 2022 Richard J. Contrada
References
Abelson, Robert P. (1995). Statistics as Principled Argument. Hillsdale, N.J.: L. Erlbaum Associates.
Cook, Thomas D, Campbell, Donald T & Peracchio, Laura. (1990). Quasi experimentation. Dunnette, Marvin D [Ed], Hough, Leaetta M [Ed]. Handbook of industrial and organizational psychology., Vol. 1, 2nd ed. Palo Alto, CA, US: Consulting Psychologists Press, US; pp. 491-576. Retrieved from http://ovidsp.ovid.com/ovidweb.cgi?T=JS&PAGE=reference&D=psyc3&NEWS=N&A….
Shadish, William R, Cook, Thomas D & Campbell, Donald T. (2002). Experimental and quasi-experimental designs for generalized causal inference. Experimental and quasi-experimental designs for generalized causal inference. Boston, MA, US: Houghton, Mifflin and Company, US; Retrieved from http://ovidsp.ovid.com/ovidweb.cgi?T=JS&PAGE=reference&D=psyc4&NEWS=N&A….
Messick, Samuel. (1995). Validity of psychological assessment: Validation of inferences from persons' responses and performances as scientific inquiry into score meaning. American Psychologist, 50, 741-749. https://doi.org/10.1037/0003-066X.50.9.741.
Cohen, J. (2016). A power primer. In A. E. Kazdin (Ed.), Methodological issues and strategies in clinical research (pp. 279–284). American Psychological Association. https://doi.org/10.1037/14805-018.